GraPL
Unsupervised Image Segmentation
2023
Partnership with Jeová Farias Sales Rocha Neto
GraPL (Graph-cuts at the Patch Level) is a patch-based unsupervised image segmentation strategy that bridges advances in unsupervised feature extraction from deep clustering methods with the algorithmic help of classical graph-based methods. We show that a simple convolutional neural network, trained to classify image patches and iteratively regularized using graph cuts, naturally leads to a state-of-the-art fully-convolutional unsupervised pixel-level segmenter. Furthermore, we demonstrate that this is the ideal setting for leveraging the patch-level pairwise features generated by vision transformer models.
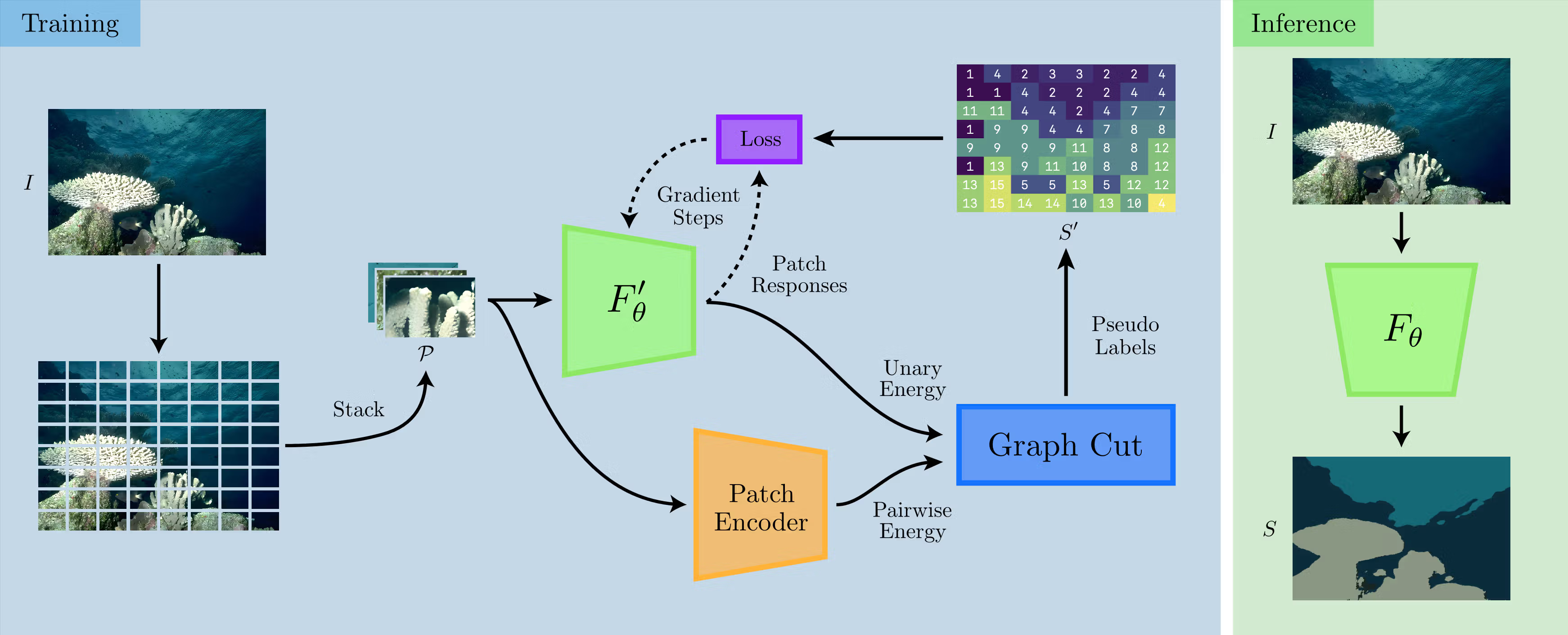
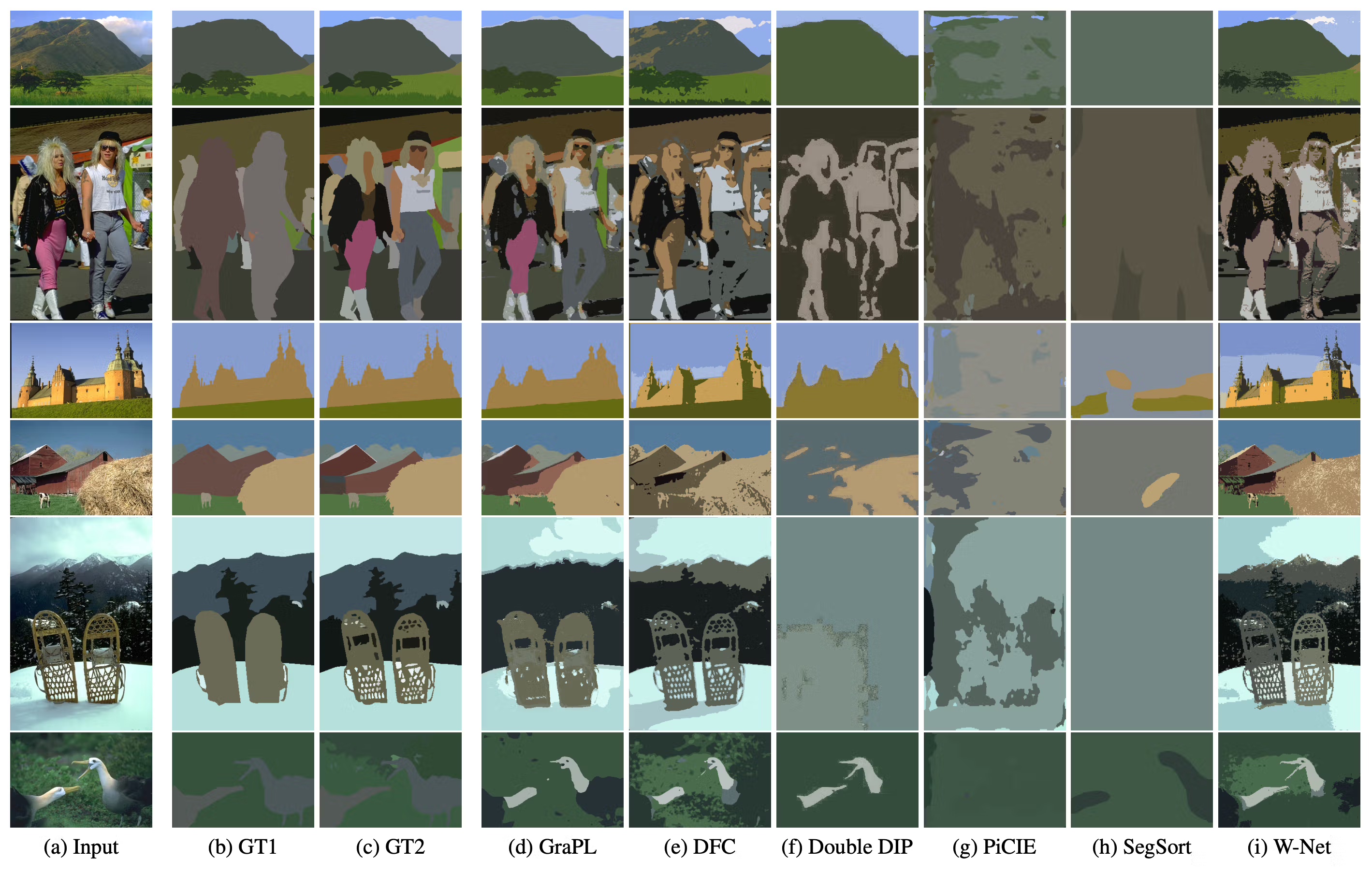